The multicloud landscape is rapidly evolving, offering businesses a wealth of resources and flexibility. However, managing workloads across diverse cloud platforms can be a complex task. Determining the optimal placement for each workload is crucial for maximising performance, minimising costs, and ensuring security. Enter Artificial Intelligence (AI) and Machine Learning (ML): powerful tools that can revolutionise multicloud workload placement.
The Challenge of Multicloud Workload Placement
In a multicloud environment, companies leverage resources from multiple cloud providers. Each cloud platform has its unique strengths and weaknesses in terms of pricing models, compute capabilities, security features, and regional availability. Choosing the “right” cloud for each workload involves a myriad of factors:
Also Read – Multi-Cloud Strategy: Your Key to Simplifying the Complex
- Cost: Optimising costs requires analysing pricing structures like pay-as-you-go models, reserved instances, discounts, and potential egress fees across different cloud providers.
- Performance: Workload performance depends on factors like CPU speed, memory allocation, network latency, and storage IOPS (Input/Output Operations Per Second). Finding the right resource combination to deliver optimal performance is key.
- Security: Security considerations include encryption standards, data residency regulations, and access control protocols. Workloads requiring high security need placement on platforms with robust security measures.
- Scalability: Workload needs can fluctuate. The chosen cloud platform must offer the flexibility to scale resources up or down quickly to meet demand.
- Compliance: Regulations within some industries dictate where data can be stored and processed. Workload placement needs to comply with these regulations.
Manually evaluating these factors for every workload across multiple cloud platforms is a tedious and error-prone process. Here’s where AI and ML come into play, offering an automated, data-driven approach to workload placement.
AI and ML: Intelligent Workload Placement
AI and ML algorithms can analyse vast amounts of data related to workloads, cloud platforms, and historical usage patterns. This allows them to develop a comprehensive understanding of workload requirements and cloud resource capabilities. By leveraging this knowledge, AI and ML can:
- Predict Resource Needs: AI can analyse workload patterns and historical data to predict future resource requirements. This enables proactive scaling to avoid performance bottlenecks and optimise costs.
- Identify Cost-Effective Options: ML algorithms can compare pricing models across multiple cloud providers based on predicted resource consumption. This helps identify the most cost-effective platform for each workload.
- Enhance Security Postures: AI can continuously monitor security threats and vulnerabilities associated with different cloud platforms. It can then recommend workloads be placed on platforms with the most robust security features aligned with specific requirements.
- Optimise Performance: ML algorithms can analyse performance metrics like CPU utilisation, network latency, and storage IOPS across various cloud configurations. This allows them to recommend the optimal combination of resources for each workload to ensure peak performance.
- Comply with Regulations: AI can integrate with regulatory compliance databases, ensuring workloads are placed on geographically appropriate cloud platforms according to data residency regulations.
Also read – How to Adopt a Multi-Cloud Strategy for Increased Flexibility and Vendor Independence
The AI/ML Workflow for Workload Placement
Here’s a simplified representation of how AI/ML can orchestrate workload placement in a multicloud environment:
- Data Collection:
- Historical workload data (CPU usage, memory utilisation, network traffic)
- Cloud platform resource information (pricing models, available configurations, security features)
- Regulatory compliance requirements
- Data Analysis and Model Training:
- AI/ML algorithms process the collected data to identify patterns and correlations between workload requirements and available cloud resources.
- This data is used to train and refine ML models that can predict optimal placement scenarios.
- Workload Characterisation:
- Each workload in the multicloud environment is analysed and characterised based on its resource needs, security requirements, and compliance constraints.
- Placement Optimisation:
- The trained ML model takes into account the characteristics of each workload and analyses the available cloud resources across different platforms. It then recommends the optimal placement for each workload based on cost, performance, security, and compliance factors.
- Continuous Monitoring and Adjustment:
- AI doesn’t sleep! The system continuously monitors workload performance and resource utilisation across different cloud platforms. It can then suggest dynamic adjustments to workload placement to maintain optimal performance and cost efficiency in a constantly changing environment.
Benefits of AI/ML-Driven Workload Placement
Implementing AI/ML for workload placement in a multicloud environment offers several advantages:
- Reduced Costs: AI/ML can identify the most cost-effective cloud platforms based on workload needs, leading to significant cost savings over time.
- Enhanced Performance: Precise workload placement based on resource requirements ensures optimal performance and avoids bottlenecks.
- Improved Security: AI can continuously monitor security risks and suggest placements that align with security best practices and regulations.
- Increased Efficiency: Automating workload placement frees up IT resources from time-consuming manual tasks, allowing them to focus on more strategic initiatives.
- Improved Agility: AI/ML enables dynamic workload placement adjustments based on changing business needs and cloud platform offerings. This allows companies to be more agile and adapt to market fluctuations.
- Data-Driven Decision Making: AI/ML eliminates guesswork from workload placement decisions. By providing data-backed recommendations, it ensures informed and objective choices.
- Reduced Risk of Vendor Lock-In: By dynamically selecting the optimal cloud platform for each workload based on current needs, AI/ML minimises reliance on a single vendor. This provides greater flexibility and control over cloud resources.
Also Read – VMware Exodus: 5 Reasons Why Apache CloudStack is the Superior Cloud Management Choice
Challenges and Considerations
While AI and ML offer immense potential for workload placement optimisation, there are challenges to consider:
- Data Quality: The accuracy of AI/ML models heavily relies on the quality and quantity of data they are trained on. Ensuring clean, comprehensive data is crucial for reliable workload placement recommendations.
- Model Development & Training: Building and maintaining accurate ML models can require expertise in data science and machine learning algorithms. Companies might need to invest in internal expertise or leverage external resources for model development.
- Integration with Existing Tools: AI/ML for workload placement needs to integrate seamlessly with existing cloud management tools and orchestration platforms. This ensures smooth adoption and reduces operational complexity.
- Explainability and Transparency: Understanding why AI/ML models recommend specific placements is crucial for building trust and ensuring alignment with business objectives.
Getting Started with AI/ML for Workload Placement
Companies interested in leveraging AI/ML for workload placement can take several steps:
- Assess Existing Infrastructure and Workloads: Evaluate the current multicloud environment and analyse data related to workload requirements and historical resource consumption.
- Identify Goals and Requirements: Define the key objectives for AI/ML-driven workload placement. Whether it’s cost optimisation, performance improvement, or enhanced security, clearly defined goals will guide the process.
- Consider External Solutions: Several cloud service providers and software vendors offer AI/ML-powered workload placement solutions. Evaluate these options based on features, pricing, and integration capabilities with existing infrastructure.
- Invest in Data Governance: Establishing strong data governance practices is crucial for maintaining accurate and high-quality data for AI/ML models.
- Start Small and Scale Up: Begin by implementing AI/ML workload placement for a pilot project in a controlled environment. Once its effectiveness is proven, it can be scaled up across the entire multicloud environment.
The Future of AI/ML in Multicloud Management
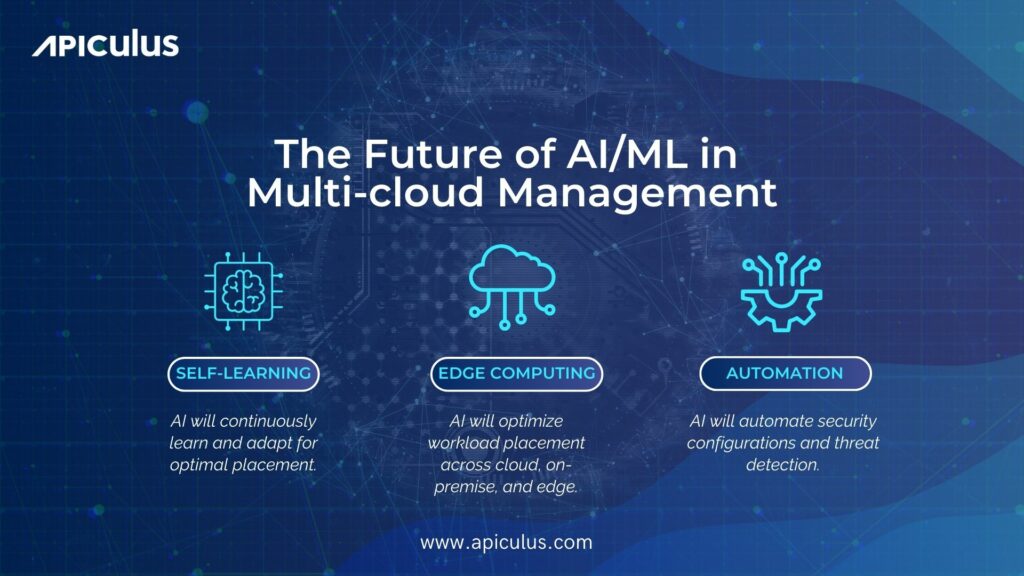
AI and ML are poised to revolutionise multicloud management. As these technologies continue to evolve, we can expect even more advanced capabilities:
- Self-Learning Workload Placement: AI models will become more sophisticated, continuously learning and adapting to changing environments, workload requirements, and cloud platform offerings. This will result in an even more dynamic and efficient workload placement process.
- Integration with Edge Computing: With the rise of edge computing, AI/ML will play a crucial role in optimising workload placement across cloud, on-premise, and edge environments.
- Security Automation: AI will be able to automate security configurations and threat detection for workloads across different cloud platforms, further enhancing multicloud security posture.
By embracing AI and ML, businesses can unlock the full potential of their multicloud environments, achieving optimal performance, cost efficiency, and security. As the multicloud landscape continues to expand, AI/ML will become an indispensable tool for managing complex and dynamic workload placements.
Also Read – On-Prem, Private, or Public? Choosing the Right Cloud Deployment Models for Your Business Needs
Apiculus: Your AI-Powered Multicloud Management Partner
Apiculus, a leading cloud management platform, offers a comprehensive solution that integrates seamlessly with your existing infrastructure and leverages AI and machine learning for intelligent workload placement across multiple cloud providers. Apiculus ingests data on your workloads, analyses cloud resource capabilities, and factors in cost structures, security requirements, and compliance regulations. It then utilises AI/ML algorithms to recommend the optimal placement for each workload within your multicloud environment.
Apiculus also provides real-time monitoring and automated adjustments to ensure your workloads consistently operate at peak performance and cost efficiency. Whether you’re looking to take your first steps towards AI-driven workload placement or seeking a comprehensive solution to manage your entire multicloud environment, Apiculus can empower you to unlock the full potential of your cloud resources.
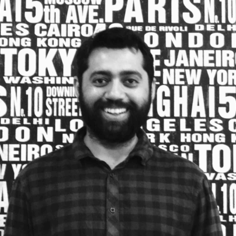
Kshitish is a ‘startup expert’ and has been involved with early stage startups, seeing various phases of growth, for more than 15 years. A specialist in Product Management, User Experience, Technology and Product Growth/Strategy, Kshitish is a seasoned entrepreneur with deep expertise in building enterprise products and horizontal/vertical SaaS. Kshitish did his PG in Product Design from NID, Ahmedabad.